Quantum Computing and Machine Learning
This blog post looks at how quantum computers can contribute to algorithms for machine learning, specifically in the training of large-scale classical models. It also looks at how fault-tolerant quantum computing could provide efficient resolutions for generic (stochastic) gradient descent algorithms.

Jens Eisert
Scientist, professor of quantum physics at @FU_Berlin and affiliated with @HZBde and @FraunhoferHHI. @jenseisert@qubit-social.xyz on Mastodon.
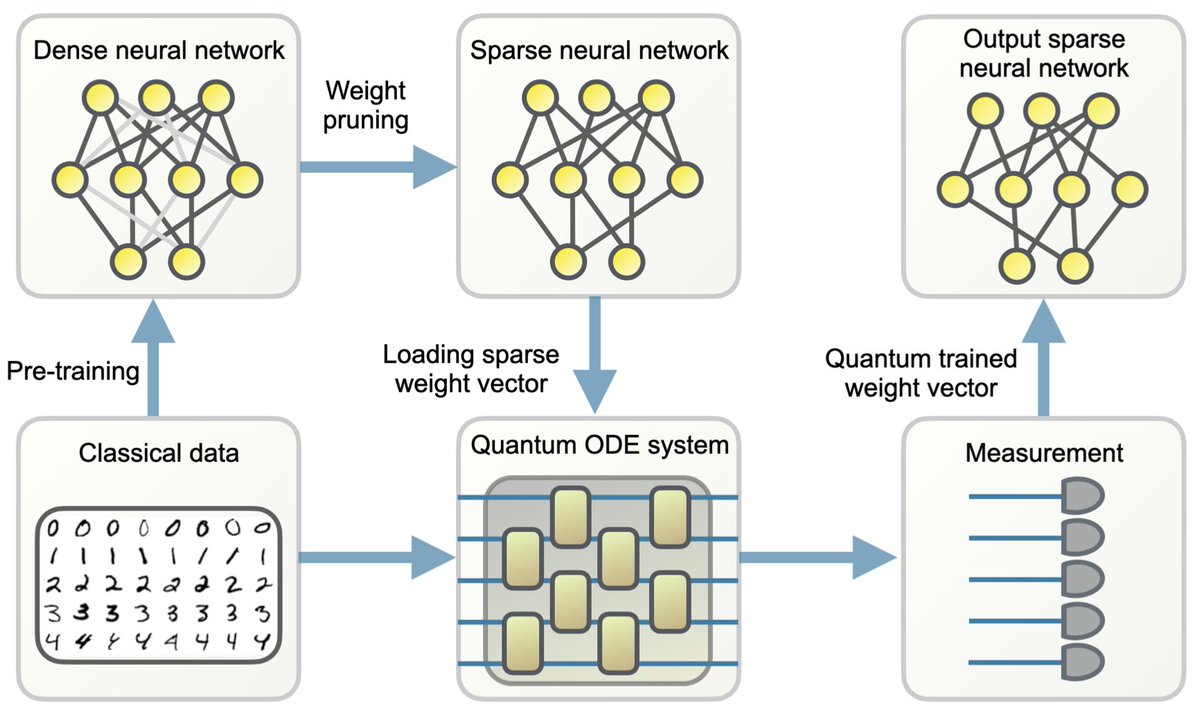
-
The biggest advantage #quantumcomputers can contribute to algorithms for #machinelearning may be in the #training of large-scale #classical models.https://t.co/MuhRvgF2OJ pic.twitter.com/9KFE9e73kR
— Jens Eisert (@jenseisert) March 8, 2023 -
In this work, we show that fault-tolerant quantum computing could possibly provide provably efficient resolutions for generic (stochastic) gradient descent algorithms, scaling as T^2 polylog(n).
— Jens Eisert (@jenseisert) March 8, 2023 -
Here n is the size of the models and T is the number of iterations in the training, as long as the models are both sufficiently dissipative and sparse.
— Jens Eisert (@jenseisert) March 8, 2023 -
Based on earlier efficient quantum algorithms for dissipative differential equations, we find and prove that similar algorithms work for (stochastic) gradient descent, the primary algorithm for machine learning.
— Jens Eisert (@jenseisert) March 8, 2023 -
We benchmark instances of large machine learning models from 7 million to 103 million parameters.
— Jens Eisert (@jenseisert) March 8, 2023 -
We find that, in the context of sparse training, a quantum enhancement is possible at the early stage of learning after model pruning, motivating a sparse parameter download and re-upload scheme. pic.twitter.com/oyw2KlS6jU
— Jens Eisert (@jenseisert) March 8, 2023 -
Our work shows that fault-tolerant quantum algorithms could potentially contribute to most state-of-the-art, large-scale machine-learning problems.
— Jens Eisert (@jenseisert) March 8, 2023 -
Warm thanks to the team of @junyuliu4, Minzhao Liu, Jin-Peng Liu, Ziyu Ye, Yuri Alexeev, and Liang Jiang for this great @UofC-@ChicagoQuantum-@qbraid_official-@argonne-@UCBerkeley-@CQE_MIT-@FUB-@FraunhoferHHI-collaboration.
— Jens Eisert (@jenseisert) March 8, 2023 -
And thanks to our funders, in particular the @BMBF (@Quantentech #Hybrid), the @BMWK (#EniQmA, #PlanQK), the @dfg_public (#CRC183), the @MunichQuantum, and the @QuantERA (#HQCC).
— Jens Eisert (@jenseisert) March 8, 2023 -
In fact, @junyuliu4 is at the #APSMarch at this moment in time. That picture was taken last week when he was spending time in Berlin. pic.twitter.com/vG8wsTV9PY
— Jens Eisert (@jenseisert) March 8, 2023